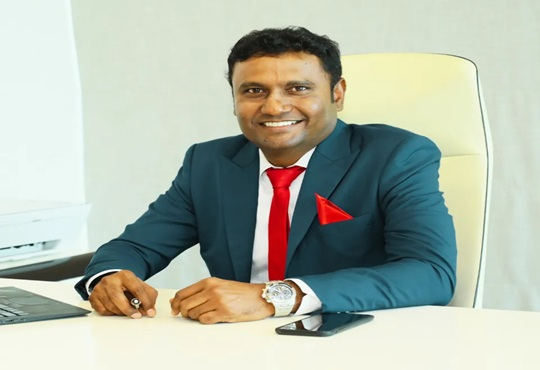
Using Artificial Intelligence to Crack the Cyber-Risk Code
Amitabh Ray, MD, Ericsson
There’s an 11-hour time difference between Bangladesh and the US, with the former ahead by almost half-a-day. February 4, 2016, was a Thursday in the US when the Federal Reserve Bank started receiving a series of requests from the Bangladesh Central Bank to pay payments totally nearly USD 1 billion to accounts individual accounts in Sri Lanka and the Philippines. Most of Bangladesh was closed for Friday prayers when the requests came into Fed.
Bangladesh’s central bank, like many other countries, maintained an account with the Fed for international transactions. Within a matter of hours around USD 101 million had been paid out when the word ‘Jupiter’, was part of the address of one of the individuals to whom the money was being paid, triggered an alarm for an entirely different reason.
By chance, Jupiter was also the name of an oil tanker and a shipping company under United States’ sanctions against Iran. That sanctions listing triggered concerns at the New York Fed and spurred it to scrutinize the payment orders more closely. It was a “total fluke” that the New York Fed did not pay out the USD 951 million requested by the hackers. The incident revealed worrying weaknesses in the global financial system, despite several irregularities in the payment requests. The messages lacked the names of “correspondent banks” – the necessary next step in the payment chain. Most of the payments were to individuals rather than institutions.
The Bangladesh heist is an insignificant sum compared to the vast amounts being lost in cybercrime around the world. The global cost of cybercrime will reach USD 2 trillion by 2019, a threefold increase from the 2015 estimate of USD 500 billion, according to “The Global Risks Report 2016,” from the World Economic Forum; and a significant portion of cybercrime goes undetected. This is particularly true in the case of industrial espionage and the heist of proprietary secrets, because illicit access to sensitive or confidential documents and data is hard to detect.
Closer home in India, over 50,300 cybersecurity incidents like phishing, website intrusions and defacements, virus and denial of service attacks were reported in 2016. Last September India’s largest bank, State Bank of India, was forced to block close to 6 lakh debit cards following a malware-related security breach in a non-SBI ATM network. Several other banks, such as Axis Bank, HDFC Bank and ICICI Bank too admitted being hit by similar cyber attacks — forcing banks to either replace or request users to change the security codes of as many as 3.2 million debit cards. The quantum of financial loss is still unknown in this case.
In all cases of cyber attacks, the hackers usually test out the system over a period of time before they strike; it is always a well-planned strategic action. While this is why they succeed it is also quite a give away that which an Artificial Intelligence based malware protecting system can detect and foil even before the attack takes place by spotting the unusualness in the patterns of transactions or ‘probes’ on the security systems being sent in by would-be hackers to install malware that will eventually give them the break-in codes.
Experts have employed machine-learning techniques, drawing on data insight to identify patterns and apply machine-readable context to events. For example, Amazon has deployed a machine learning solution, based on a unique algorithm that can predict customer-spending habits. In the security world, similar machine-learning systems are used for anomaly detection. This means that a model is defined and positioned as normal. If an outlier is detected that differs from this model, it is considered to be an anomaly. Importantly, the ‘normal’ model is not static. As more data is added, the definition of ‘normal behavior’ continues to evolve and reflect the environment to ensure it remains accurate and up-to-date.
This also means that if unknown threats are assessed against the model, they are more likely to be identified because they do not fit the expected behavioral pattern. Security researchers at MIT have developed a new Artificial Intelligence-based cyber security platform, called 'AI2,' which has the ability to predict, detect, and stop 85 percent of Cyber Attacks with high accuracy.
AI-powered anti-malware cyber security solutions are different from anti-virus which reactively detects viruses or malware. Artificial Intelligence security solutions proactively finds out the possibilities of an attack happening even before it has taken place by applying machine learning techniques to analyze the behavioral patterns of the would-be hackers. In the Bangladesh instance, this would be the payment requests not having the names of the correspondent banks or that the same requests had earlier come in an unformatted manner but were later corrected.
Whatever method cyber criminals use, they are bound to create some kind of a pattern that will be creating a digital ripple which only the ultra-sensitive radars of Artificial Intelligence which has been used to train the computers can detect. But the same technologies that improve corporate defenses could also be used to attack them. Take phishing; it's the simplest method of cyber attack available and there are schemes on the dark web which put all the tools required to go phishing into anyone's hands. Like in the physical world of law enforcers and criminals, in the cyberspace too it will be a race of who stays a step ahead in the game.
CIO Viewpoint
Why Foolproof Facial Recognition Is Key Against...
By Joseph Sudheer Thumma, Global CEO & MD, Magellanic Cloud
National Technology Day 2025: Powering Progress...
By CIOTech Outlook Team
Aligning IT Roadmap with Business Objectives: A...
By Subhash singh Punjabi, CISO & Head Enterprise Architecture, Deepak Fertilisers & Petrochemicals Corporation Ltd
CXO Insights
How to Make Risk Heatmaps More Effective
By Shankar Bhaskaran, Managing Director – India, MetricStream
Building Resilience through Robust Operational...
By Shankar Bhaskaran, Managing Director – India, MetricStream
Project Management and Risk Planning